Quantitative social norms favor the evolution of cooperation
What does it mean to be a good person? How do we best form an opinion about others in our community, and how do we interact with them based on this opinion? The field of indirect reciprocity approaches these fundamental questions mathematically. It explores how people form reputations, and how social norms emerge, with the help of game theory.
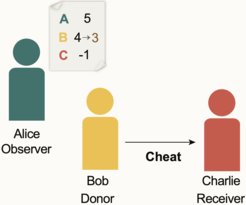
Much previous research on indirect reciprocity looks at social norms that can lead to cooperation in a society. There are eight well-known successful social norms, called the “leading eight”. When individuals use these norms, they classify each other either as “good” or “bad”. They then decide with whom to cooperate based on these labels. Such simple moral norms can lead to interesting dynamics. For example, when Alice starts with a good reputation, but then cheats against Bob, other group members may start to see her in a bad light. In this way, reputations in a community can change in time, depending on how people behave and which norms they apply. When everyone uses one of the leading eight norms, the community tends to be fully cooperative, and cheaters cannot take over. Much of this previous work, however, assumes that all relevant information about individual reputations is public. As a result, opinions are perfectly synchronized – if Alice deems Bob as good, everyone else in her community agrees. Yet, in many real-world scenarios, people only have access to information that is incomplete or noisy. They might not observe everything in their community, or they might misinterpret some of their observations. Moreover, people don’t necessarily share all their information or opinions with one another. As a result, even if Alice deems Bob as bad, Charlie might think otherwise. Once information is private and noisy, previous work suggests that none of the leading eight are able to stabilize cooperation. The problem is that initial disagreements in opinions can proliferate. Once Alice and Charlie disagree on Bob, they might also start to disagree on each other’s reputation.
Avoiding the accumulation of different opinions
This problem is now addressed in a new article by researchers from KAIST, IST Austria, and the Max Planck Institute of Evolutionary Biology. The researchers set out to explore just how reputation systems can avoid accumulating disagreements. To this end, they studied social norms that allow individuals to have more complex reputations than just “good” and “bad”. Instead, individuals use numerical scores to track each other’s reputations. For example, Alice may have a score of 5, whereas Bob is a 3. These scores can then again change in time, depending on someone’s interactions and on the community’s social norms. Reputations below a certain threshold are then overall classified as “bad”, while scores equal to or above the threshold are classified as “good”. This model naturally leads to a more fine-grained way of describing “moral goodness”. When people use this kind of quantitative reputation system, there is more leeway for single mistakes or missed observations. For example, if Alice assigns a score of 4 to Bob, and misinterprets one of his actions in a bad way, she will decrease his score only by 1, which leads to her deeming Bob as a 3. If the threshold is set to the neutral 0, that means she still judges Bob as overall “good”, despite her lapse in observation.
The researchers found that such quantitative assessments can dramatically increase the robustness of a moral system. In fact, their computer simulations show that quantitative reputations allow four of the leading eight norms to be robust against cheaters and blind altruists even if decisions are based on imperfect and noisy information. This observation can be formalized. The team proved that when populations adopt one of these four norms, they are more likely to recover from initial disagreements, and this recovery happens more quickly.
Cooperative norms beyond the leading eight
“These results demonstrate how fine-grained reputation systems, and by extension a modified definition of ‘goodness’, can help cooperation under conditions when black-and-white ‘partisan’ thinking fails”, lead author Laura Schmid explains. “Polarization by only assuming others to be good or bad, nothing in between, can significantly hurt a community. It takes some buffer for mistakes.” Surprisingly, however, these findings also suggest that reputation systems should not become overly fine-grained. Instead, there is an optimal scale at which behavior should be assessed. The researchers note that this does not only explain some key features of naturally occurring social norms, but it also has interesting implications for the optimal design of artificial reputation systems. “In this way, our study may serve as a starting point for future challenges, by exploring cooperative norms beyond the leading eight”, Schmid explains.