Current Research Projects
The main research theme of our group is the evolution of translation in bacteria [1-4]. We currently have projects on the evolution of tRNA gene sets and translation termination factors. We are also interested in bacterial chromosome architecture, and its effects on evolutionary flexibility [5].
Translation 1: the evolution of bacterial tRNA sets
Even though all tRNA sets serve broadly the same function – decoding 61 codons into 20 amino acids – tRNA gene complements differ considerably between organisms. For example, bacterial tRNA sets typically contain 28-46 types of tRNA, encoded by 28-120 genes. The evolutionary and molecular mechanisms by which different tRNA sets emerge have been of long-standing interest.
Our group has recently provided two direct observations of a bacterial tRNA gene set evolving in real time [1,2]. We began by perturbing the tRNA set of the model bacterium, Pseudomonas fluorescens SBW25. Specifically, we deleted a serine tRNA gene (giving strain del-serCGA), and a four-gene glutamic acid/glycine tRNA locus (giving strain del-EGEG). The resulting growth defects were compensated during a 21-day serial transfer evolution experiment. Compensation occurred via duplication of a remaining tRNA gene (serTGA or glyGCC). The tRNA gene deletions caused a shortage of tRNA-Ser or tRNA-Gly, generating a growth defect that was relieved by duplicating remaining tRNA genes to (re)elevate tRNA-Ser/Gly levels.
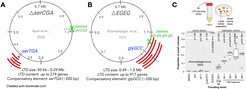
Instead of simply duplicating the ~200 bp tRNA gene, compensated mutants carry a large, tandem duplication (LTD). The LTDs are up to ~1 Mb in size and occur in two distinct genomic regions (Fig. 1A-B). LTDs have a multitude of effects, including (i) changing the copy number of hundreds of genes, (ii) modifying chromosomal architecture, and (iii) adding considerable extra DNA for replication. Nonetheless, the net effect of each LTD is positive; any negative effects are outweighed by the advantage of gaining a tRNA gene. Finally, the LTDs are adaptive but mechanistically unstable; in an overnight culture founded by an LTD-carrying strain, ~3% to 34 % of cells lose the duplication (Fig. 1C).
These observations prompted us to ask the question: what becomes of the adaptive-but-unstable large duplications over longer evolutionary time scales? Currently, we are extending the serial transfer evolution experiment to 100 days to answer this question.
Translation 2: the evolution of release factor control
Translation termination involves release factors (RFs). In bacteria, the three stop codons are together recognized by two RFs (RF1 and RF2), the expression of which is well-regulated. We are interested in the evolvability of a highly conserved, yet apparently disposable, autoregulatory system for the expression of RF2 (encoded by the prfB gene). The autoregulatory system functions as follows: (i) when RF2 levels are high, the internal stop is recognised (by RF2 itself), truncating RF2 expression, while (ii) at lower RF2 levels, the stop codon is bypassed by ribosomal frameshifting, leading to full-length RF2 expression. The frameshift is promoted by several conserved features of the ~16 nucleotides around the internal stop (the ‘PRF site’; Fig. 2).
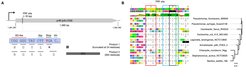
The prfB internal stop is evolutionarily conserved among bacteria, but has nevertheless been lost in some clades (and can be removed in the lab with few effects). With the ultimate aim of providing insight into the evolutionary history of RF2 autoregulation, we are investigating the evolvability of the P. fluorescens SBW25 PRF site.
Genome architecture: evolutionary flexibility of bacterial chromosomes
In bacteria, essential genes tend to be located around the origin of replication (ori), while non-essential genes are found around the replication terminus (ter). This compartmentalization has been predicted to lead to faster evolution around ter. My group aims to empirically test this hypothesis. In a previous (unrelated) project, we isolated a P. fluorescens SBW25 strain with a large deletion across ter. We are currently using this strain to explore SBW25 genome organization.
Literature
1. Ayan, Park, Gallie. 2020. eLife 9:e57947.
2. Khomarbaghi, Ngan, Ayan, Lim, Dechow-Seligmann, Nandy, Gallie. 2024. Nucleic Acids Res. 52(5):2446-2462.
3. Raval, Ngan, Gallie, Agashe. 2023. eLife 12:e81005.
4. van der Gulik, Egas, Kraaijeveld, Dombrowski, Groot, Spang, Hoff, Gallie. 2023. RNA Biol. 12:e81005.
5. Mukherjee, Dechow-Seligmann, Gallie. 2022. Mol. Micro. 117(2):394-410.